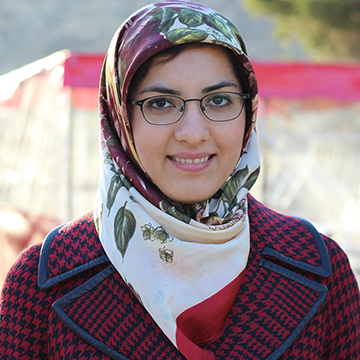
PhD Candidate
UC Berkeley
Areas of Interest
- Artificial Intelligence
- Computer Vision
- Machine Learning
Poster
Semantic Bottleneck Scene Generation
Abstract
Coupling the high-fidelity generation capabilities of label-conditional image synthesis methods with the flexibility of unconditional generative models, we propose a semantic bottleneck GAN model for unconditional synthesis of complex scenes. We assume pixel-wise segmentation labels are available during training and use them to learn the scene structure through an unconditional progressive segmentation generation network. During inference, our model first synthesizes a realistic segmentation layout from scratch, then synthesizes a realistic scene conditioned on that layout through a conditional segmentation-to-image synthesis network. When trained end-to-end, the resulting model outperforms state-of-the-art generative models in unsupervised image synthesis on two challenging domains in terms of the Frechet Inception Distance and perceptual evaluations. Moreover, we demonstrate that the end-to-end training significantly improves the segmentation-to-image synthesis sub-network, which results in superior performance over the state-of-the-art when conditioning on real segmentation layouts.
Bio
Samaneh Azadi is a Ph.D. candidate in Computer Science at UC Berkeley, advised by Prof. Trevor Darrell. Her research has been focused on machine learning and computer vision particularly in creative image generation through structural generative adversarial modeling. She has been awarded the Facebook Graduate Fellowship and the UC Berkeley Graduate Fellowship and was named a Rising Star in EECS in 2019. She has spent time as a research intern at Google Brain and Adobe Research. Samaneh is co-organizing the NeurIPS 2020 Workshop on Machine Learning for Creativity and Design and has co-organized the Women in Computer Vision Workshop (WiCV) twice at CVPR 2016 and CVPR 2017.+M4