[an error occurred while processing this directive]
[an error occurred while processing this directive]
[an error occurred while processing this directive]
[an error occurred while processing this directive]
Research Scientist
[an error occurred while processing this directive]
Google Research
[an error occurred while processing this directive]
PhD '17 University of California, Berkeley
[an error occurred while processing this directive]
[an error occurred while processing this directive]
[an error occurred while processing this directive]
Information, Data, Network, and Communication Sciences
Artificial Intelligence
[an error occurred while processing this directive]
A Scientific Approach To Bridging Theory & Practice in Machine Learning
[an error occurred while processing this directive]
Deep neural networks are a rich, versatile class of models that can be applied across many domains, but our understanding of their function, design, and limitations in modern settings lags behind, thereby hindering safe deployment and future research progress. I’ll overview research in which I take a scientific approach to understanding deep neural networks, guided closely by empirical work and practice. I’ll touch on my prior work that has given rise to useful connections between overparameterized deep neural networks, kernel methods, and Gaussian processes. I’ll then focus on a specific illustrative result studying the gradient-descent dynamics of deep neural networks at large learning rates. I’ll discuss how the choice of learning rate is a crucial factor naturally classifying the dynamics of deep networks into two phases, separated by a sharp phase transition, and the implications of this for model performance. Overall, I hope to illustrate the merits of a scientific approach combining theory and empirics towards shedding light on complex, multi-component machine learning systems.
[an error occurred while processing this directive]
Yasaman Bahri is a Research Scientist at Google on the Brain Team. Her present research program is to understand the workings of machine learning systems, with a current focus on deep learning, and bridge the gap between practice and theory. She uses a combination of theoretical analysis and empirical investigation to elucidate mechanisms and diagnose limitations, with the goal of designing more reliable and performant systems. She has broad multi-disciplinary interests that span much of machine learning as well as neighboring fields. She was trained as a theoretical condensed matter physicist, in the area of strongly correlated systems, and received her Ph.D. in Physics from UC Berkeley in 2017. She is a recipient of the NSF Graduate Fellowship and Departmental Citation Award in Physics from UC Berkeley.
[an error occurred while processing this directive]
Personal home page
[an error occurred while processing this directive]
[an error occurred while processing this directive]
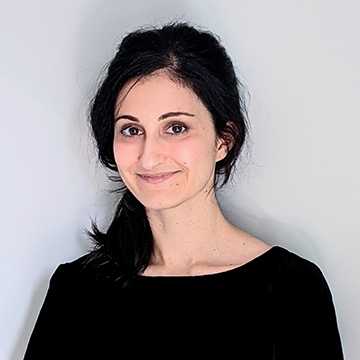