[an error occurred while processing this directive]
[an error occurred while processing this directive]
[an error occurred while processing this directive]
[an error occurred while processing this directive]
Postdoctoral Scholar
[an error occurred while processing this directive]
Stanford University
[an error occurred while processing this directive]
PhD '19 Rensselaer Polytechnic Institute
[an error occurred while processing this directive]
[an error occurred while processing this directive]
[an error occurred while processing this directive]
Artificial Intelligence
[an error occurred while processing this directive]
Radiology-Pathology Fusion for Automated Prostate Cancer Detection
[an error occurred while processing this directive]
Magnetic Resonance Imaging (MRI) is widely used for screening and staging prostate cancer. However, the subtle differences between benign and cancerous tissue on prostate MRI results in an alarming variability in radiologist interpretation. Automated methods for prostate cancer detection on MRI can help standardize radiologist interpretation and assist in early diagnosis and treatment planning. However, existing automated methods mostly use inaccurate ground truth labels with MRI features alone, ignoring disease pathology characteristics observed on resected tissue. Here, we present CorrSigNet, a radiology-pathology fusion approach for detecting and localizing prostate cancer on MRI. CorrSigNet learns from patients with pathology after surgery, and then applies the learning to patients without pathology. This makes it clinically useful in prostate cancer detection, biopsy targeting and treatment planning. CorrSigNet uses registered pre-operative MRI and post-operative whole-mount histopathology images from prostate surgery patients to derive accurate ground truth labels and learn correlated features from the two modalities. CorrSigNet then uses the learned correlated features together with original MRI intensities in a deep learning framework to detect cancer on MRI. CorrSigNet outperforms existing prostate cancer detection methods, achieving a pixel-level AUC of 0.86 and a lesion-level AUC of 0.96 +- 0.07.
[an error occurred while processing this directive]
Dr. Indrani Bhattacharya is a Postdoctoral Scholar at Stanford University
where she holds a joint appointment at the Laboratory for Integrative Personalized Medicine and the Urologic Cancer Innovation Laboratory. Prior to joining Stanford, Dr. Bhattacharya received her Ph.D. and M.S. in Electrical Engineering from Rensselaer Polytechnic Institute (RPI), NY. Her research interests are in machine learning, computer vision, and multimodal data analytics applied to several interdisciplinary real-world problems in precision medicine, biomedical image processing, human-centered computing, and social signal processing. Her current postdoctoral research focuses on multimodal and multi-scale data fusion for developing automated methods for cancer detection. Her doctoral research focused on the development of multi-sensor fusion algorithms for estimating and analyzing human behavior in group interactions. Dr. Bhattacharya held a research intern position at IBM Research briefly during her graduate studies. She has been the recipient of multiple awards, including the MICCAI 2020 NIH award, the Founder’s Award of Excellence from RPI, best poster, perfect pitch and travel awards from DoE, NSF, NIH, C3E Initiative, Women in Computer Vision, and RPI. She completed her Bachelor’s in Electrical Engineering from Jadavpur University, India and worked as a Project Engineer in Indian Oil Corporation Limited before transitioning to her graduate education.
[an error occurred while processing this directive]
Personal home page
[an error occurred while processing this directive]
[an error occurred while processing this directive]
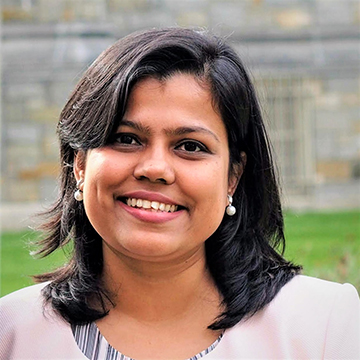