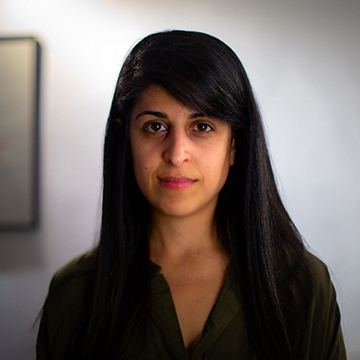
PhD Candidate
Princeton University
Areas of Interest
- Artificial Intelligence
- Theory
- Algorithms for Machine Learning
Poster
New directions in Boosting
Abstract
Boosting is a widely used machine learning approach based on the idea of efficiently aggregating weak learning rules into a strong one. Boosting was first studied in the seminal work of Freund and Schapire, in the context of PAC learning, which includes the celebrated Adaboost algorithm as well as many other algorithms. The theory of boosting has been studied extensively, and has transformed machine learning across a variety of applications, leading to a tremendous practical success. In recent years, the fruitful connection between boosting and online learning methods has yielded new observations and applications in which boosting was not previously explored. This work builds on and is inspired by these contributions, and expands the application of boosting algorithms to the online agnostic setting, and to the bandit setting in online learning.
Bio
Nataly Brukhim is a PhD student in the Department of Computer Science at Princeton University, advised by Professor Elad Hazan. Her research focuses on theory and algorithms for machine learning and, in particular, Boosting algorithms and online learning. She is also a student researcher at Google AI Princeton. Prior to this, she was a research intern at Cornell University. She received a B.Sc and a M.Sc degrees in Computer Science (graduating cum laude), from Tel Aviv University.