[an error occurred while processing this directive]
[an error occurred while processing this directive]
[an error occurred while processing this directive]
[an error occurred while processing this directive]
PhD Candidate
[an error occurred while processing this directive]
Carnegie Mellon University
[an error occurred while processing this directive]
[an error occurred while processing this directive]
[an error occurred while processing this directive]
[an error occurred while processing this directive]
Artificial Intelligence
Natural Language Processing
Computer Vision
Deep Learning
Generation
[an error occurred while processing this directive]
Effective Controllability of Multimodal Narrative Intelligence
[an error occurred while processing this directive]
Anthropomorphic narrative generation in natural language in the form of stories, procedures, etc., has been a long-standing dream of artificial intelligence. Working towards this goal brings forth the need to adhere to the innate human characteristics of narratives. This opportunity urges us to model heterogeneous surrounding contexts from vision, auditory, and language signals. Through the course of my Ph.D. thus far, I proposed an anchoring framework to improve three fundamental components of 'Controllable Multimodal Natural Language Generation'. Specifically, I propose a 2D anchoring framework to distill the scope of this multi-dimensional problem to perform an attributable evaluation. The first dimension is discrete with the three narrative properties: (1) content i.e, relevance, (2) structure i.e, coherence and (3) surface form realization i.e, expression, seeking input from visual and textual modalities. The second dimension of modeling approaches determines the annotation complexity between supervised and unsupervised techniques giving rise to ‘locally’ (word level) and ‘globally’ (narrative level) conditioned training objectives. Contextualizing the effectiveness of this novel framework for each of the 3 properties, I present (1) Content: In situated multimodal contexts, relevance is the concept of the elements in one modality being connected to the other modality that makes this context complementary and informative. I present a simple yet effective technique of visual infilling with curriculum learning as a global objective and hierarchical skeleton-based learning as a local objective to generate visual stories and procedures. In order to improve the controllability, I also introduced a dual staged model that extracts a skeleton first to denoise the content in an image caption. (2) Structure: The alignment of description in language to the corresponding visual inputs is crucial to generate a logical and coherent narrative. I present a scaffolding technique as a local objective to extract a structural layout from vast amounts of unsupervised text to incorporate structure into cooking recipes generated from images. As a global objective, I proposed new techniques to improve reordering of sentences at various granularities. (3) Surface Form Realization: The crux of naturalness to automatic generation comes by incorporating individualized and personalized ways of expressing the same content. I worked on a dual staged GAN model to improve the generation of mixed-language text with a global sentence level objective, and speech with a local word-level objective.
[an error occurred while processing this directive]
Khyathi Chandu is a Ph.D. candidate at the Language Technologies Institute at Carnegie Mellon University. She received her bachelors in Computer Science from IIIT Hyderabad. Her primary research interests lie in the area of text generation and multimodal machine learning, and she has also worked in multilingual processing, question answering, and summarization. She has worked in deep learning techniques that explicitly and implicitly impact long-form text generation from vision and language modalities. She enjoys teaching, providing guidance, and has also been awarded Outstanding Reviewer at ACL 2020. Her team also won the BioAsq challenge in 2018. She is an S.N.Bose scholar and has been shortlisted for Apple Fellowship. She also serves as a Diversity and Inclusion co-chair at ACL 2020 and ACL 2021, and is an organizer of WiNLP in 2020 and 2021. She was also awarded the Best All-rounder Student during her undergraduation and the Best Outgoing Student prior to that.
[an error occurred while processing this directive]
Personal home page
[an error occurred while processing this directive]
[an error occurred while processing this directive]
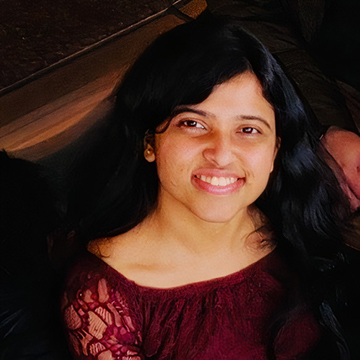