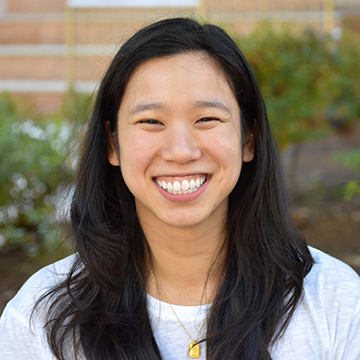
PhD Candidate
Massachusetts Institute of Technology
Areas of Interest
- Artificial Intelligence
Poster
Clustering Censored Multivariate Time-Series for Disease Phenotyping
Abstract
Unsupervised learning seeks to uncover sub-structure in data. However, different kinds of noise may impede useful structure discovery from real-world data. Motivated by the application of disease phenotyping, we present a deep generative model to infer disease subtype and patient alignment from longitudinal and observational patient data. Existing disease progression models often incorrectly assume patient data are already aligned by disease stage. In reality, we may see patients at the time of diagnosis who are at different stages of the disease --- both late and early. This can arise, for example, due to differences in when patients seek medical care and such discrepancy can confound unsupervised learning algorithms. We demonstrate accurate, stable, and interpretable results on synthetic data that outperform several benchmarks. On two clinical datasets, our model reconstructs known phenotypes and trajectories and suggests areas for future clinical research.
Bio
Irene Chen is a computer science PhD student at MIT, advised by David Sontag. Her research focuses on machine learning methods to improve clinical care and deepen our understanding of human health, with applications in areas such as heart failure and intimate partner violence. Her work has been published in both machine learning conferences (NeurIPS) and medical journals (Nature Medicine, AMA Journal of Ethics), and covered by media outlets including MIT Tech Review, NPR/WGBH, and Stat News. Prior to her PhD, Irene received her AB in applied math and SM in computation engineering from Harvard University.