[an error occurred while processing this directive]
[an error occurred while processing this directive]
[an error occurred while processing this directive]
[an error occurred while processing this directive]
PhD Candidate
[an error occurred while processing this directive]
University of California, Berkeley
[an error occurred while processing this directive]
[an error occurred while processing this directive]
[an error occurred while processing this directive]
[an error occurred while processing this directive]
Artificial Intelligence
Control, Intelligent Systems, and Robotics
Machine Learning
[an error occurred while processing this directive]
Safe and Robust Perception-Based Control
[an error occurred while processing this directive]
Machine learning provides a promising path to distill information from high dimensional sensors like cameras---a fact that often serves as motivation for merging learning with control. This talk aims to provide rigorous guarantees for systems with such learned perception components in closed-loop. Our approach is comprised of characterizing uncertainty in perception and then designing a robust controller to account for these errors. We provide performance guarantees in the linear setting and safety guarantees in the nonlinear setting, illustrating how trade-offs arise from limitations of the training data. Joint work with Ryan Cosner, Nikolai Matni, Ben Recht, Andrew J. Taylor, and Vickie Ye.
[an error occurred while processing this directive]
Sarah is a PhD candidate in the EECS department at UC Berkeley, working with Ben Recht. She is funded by a Berkeley fellowship and the NSF graduate research fellowship. She has a MS in EECS from Berkeley and a BSE in electrical engineering and math from the University of Pennsylvania.
Sarah is interested in interplay between optimization, machine learning, and dynamics in real-world systems. Her research focuses on developing principled data-driven methods for control and decision-making, broadly categorized into two thrusts: guaranteeing safety in feedback control and ensuring values in social-digital systems. This work is grounded in and inspired by collaborative projects in robotics, recommendation systems, and developmental economics.
Sarah is a founding member of Graduates for Engaged and Extended Scholarship in computing and Engineering (GEESE), a transdisciplinary student group aiming to give graduate students a constructive place to reflect on issues of society and technology.
[an error occurred while processing this directive]
Personal home page
[an error occurred while processing this directive]
[an error occurred while processing this directive]
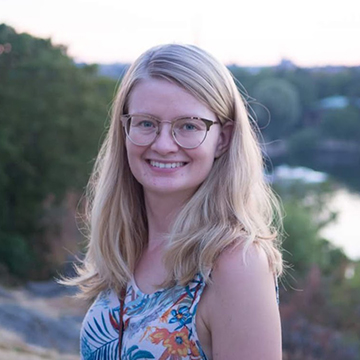