[an error occurred while processing this directive]
[an error occurred while processing this directive]
[an error occurred while processing this directive]
[an error occurred while processing this directive]
PhD Candidate
[an error occurred while processing this directive]
University of Colorado Boulder
[an error occurred while processing this directive]
[an error occurred while processing this directive]
[an error occurred while processing this directive]
[an error occurred while processing this directive]
Artificial Intelligence
Theory
Algorithmic Bias
[an error occurred while processing this directive]
An Embedding Framework for Consistent Convex Surrogates
[an error occurred while processing this directive]
We formalize and study the natural approach of designing convex surrogate loss functions via embeddings for problems such as classification or ranking. In this approach, one embeds each of the finitely many predictions (e.g. classes) as a point in R^d, assigns the original loss values to these points, and "convexifies" the loss in between to obtain a surrogate. We prove that this approach is equivalent, in a strong sense, to working with polyhedral (piecewise linear convex) losses. Moreover, given any polyhedral loss L, we give a construction of a link function through which L is a consistent surrogate for the loss it embeds. We go on to illustrate the power of this embedding framework with succinct proofs of consistency or inconsistency of various polyhedral surrogates in the literature.
[an error occurred while processing this directive]
Jessie Finocchiaro is 4th year of my PhD in the CS Theory group at CU Boulder, working with Drs. Rafael Frongillo and Bo Waggoner. Her research interests intersect Theoretical Machine Learning, Algorithmic Game Theory, and Computational Economics. In particular, she is interested in decision-making in the midst of uncertainty, how the questions we ask affect what we learn [from people, machine learning algorithms], and how this uncertainty affects people. She is also a 2019 National Science Foundation Graduate Research Fellow.
[an error occurred while processing this directive]
Personal home page
[an error occurred while processing this directive]
[an error occurred while processing this directive]
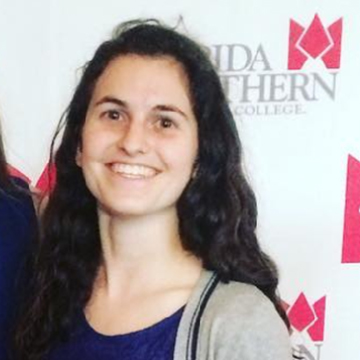