[an error occurred while processing this directive]
[an error occurred while processing this directive]
[an error occurred while processing this directive]
[an error occurred while processing this directive]
PhD Candidate
[an error occurred while processing this directive]
Cornell University
[an error occurred while processing this directive]
[an error occurred while processing this directive]
[an error occurred while processing this directive]
[an error occurred while processing this directive]
Theory
Market Design
Algorithmic Game Theory
Revenue Management and Pricing
Online Learning
[an error occurred while processing this directive]
Satisficing Search and Algorithmic Price Competition
[an error occurred while processing this directive]
The rising prevalence of algorithmic pricing in industry calls for a closer study of market outcomes that arise when these algorithms interact in a competitive environment. In this paper, we study the impact of boundedly rational customer choice on the landscape of joint demand curves in competition, and its consequences on the properties of competing pricing algorithms derived from the online convex optimization (OCO) framework. In particular, we study price competition in a duopoly under a new customer behavior model motivated by online platforms offering perfectly substitutable services, e.g., ride-hailing. In this model, a customer samples the firms in an idiosyncratically preferred order until she finds one that is priced below her willingness-to-pay.
We exhaustively characterize the equilibrium profile of the resulting pricing game when customers' willingness-to-pay distribution has a monotone hazard rate. We show that these games are frequently plagued by a particular strictly-local Nash equilibrium, in which the price of the firm with a smaller market share is only a local best-response to the competitor's price, when a globally optimal response with a potentially unboundedly higher payoff is available. Through numerical experiments, we show that price dynamics resulting from distributed online gradient descent may often converge to this undesirable outcome, and as a result cause the smaller firm to incur linear regret relative to her best-response price. Our results thus suggest that algorithms that adaptively rely on local information to learn good pricing decisions, {despite faring well in monopolistic settings}, may suffer from serious drawbacks in competitive environments. We finally propose and test a practical resolution of this concern in the context of our model.
[an error occurred while processing this directive]
Chamsi Hssaine is a fifth-year graduate student in the School of Operations Research and Information Engineering at Cornell University, where she is advised by Professor Siddhartha Banerjee. She obtained her B.S. in Operations Research and Financial Engineering (ORFE) from Princeton University in 2016, graduating magna cum laude. Her research lies at the intersection of theoretical computer science, economics and operations research, and focuses on the algorithmic design of modern marketplaces. She is a recipient of the Princeton ORFE department’s Kenneth H. Condit Prize for leadership through academic achievement and community service. Her paper “Satisficing Search and Algorithmic Price Competition” was recently selected for the Stanford Graduate School of Business Rising Scholars Conference
[an error occurred while processing this directive]
Personal home page
[an error occurred while processing this directive]
[an error occurred while processing this directive]
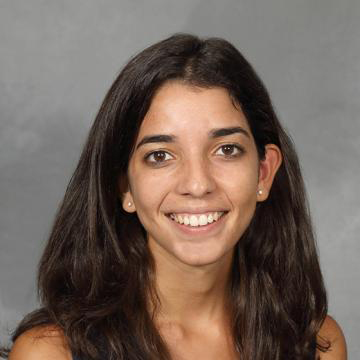