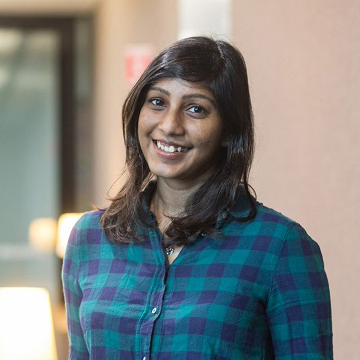
Postdoctoral Researcher
Singapore Management University
PhD '19 Singapore Management University
Areas of Interest
- Artificial Intelligence
- Cyber-Physical Systems and Design Automation
- Ubiquitous Computing
Poster
The Case for Collaborative Sensing on the Edge
Abstract
A variety of physical environments, including smart cities and tactical battlefield networks are increasingly being instrumented with large numbers of resource-constrained sensors and IoT devices (e.g., cameras, microphone arrays and environmental sensors). A rising recent trend involves executing inferencing pipelines (to perform increasingly complex tasks, such as object recognition or target localization), in-situ and in real time, at such edge nodes. There are two salient features associated with these trends: (1) Sensors are often deployed with varying degrees of redundant coverage--e.g., cameras in buildings often have partially overlapping fields of view, implying that their sensed data are implicitly spatiotemporally correlated, and (2) inferencing increasingly involves the execution of computationally prohibitive machine learning (ML) pipelines (e.g., CNNs for image-based object detection). Executing such deep neural networks (DNNs) gives rise to well-known throughput bottlenecks and prohibitive energy consumption. I introduce and explore the paradigm of Collaborative Deep Intelligence that exploits sensor multiplicity (e.g., a group of networked cameras with overlapping views) for performance benefits such as improved accuracy with minimal overhead of latency. This work is motivated by a fundamental research question: to enable execution on mobile/embedded devices, is it possible to rely on collaboration to replicate the accuracy of very-deep, but high accuracy neural networks while incurring the computational expense only of shallower, older DNNs (with concomitant latency and energy benefits)?
Bio
Kasthuri is a Research Fellow at the Living Analytics Research Center, Singapore Management University (SMU). Recently, she completed her doctoral research at SMU in the areas of socio-physical sensing and pervasive computing, advised by Prof. Archan Misra. Her work explores solutions to real-world urban problems cutting across applied machine/deep learning, edge computing, indoor/outdoor mobility modelling, network science, urban science and marketing science, and has featured at several top-tier conferences and journals (UbiComp/IMWUT, MobiSys, SenSys, CIKM, ACM TOIT). Recently, she was named a finalist for the Gaetano Borriello Outstanding Student Award at UbiComp 2019, and is a past recipient of the Google Women Techmakers Scholarship (APAC, 2017), the SMU Multidisciplinary Doctoral Fellowship (2018), SMU-CMU LARC Exchange Fellowship (2016) and the A*STAR Graduate Scholarship (2014-2018).