[an error occurred while processing this directive]
[an error occurred while processing this directive]
[an error occurred while processing this directive]
[an error occurred while processing this directive]
PhD Candidate
[an error occurred while processing this directive]
University of California, Los Angeles
[an error occurred while processing this directive]
[an error occurred while processing this directive]
[an error occurred while processing this directive]
[an error occurred while processing this directive]
Artificial Intelligence
Biosystems and Computational Biology
[an error occurred while processing this directive]
Electronic health record signatures identify undiagnosed patients with Common Variable Immunodeficiency Disease
[an error occurred while processing this directive]
As the immune system is intertwined with nearly all organs and tissues, the clinical presentation of rare immune diseases intersects with virtually every medical specialty, causing the fragmentation of patients across multiple clinical sub-specialties, causing a significant delay in initiating clinical care for Common Variable Immunodeficiency (CVID) patients . Our risk score algorithm, PheNet, focuses on identifying a set of EHR-derived features that characterize CVID with high sensitivity and specificity as well as a feature weighting scheme. The feature set is defined by billing code information from labeled CVID patients as well as the symptoms reported in the Online Mendelian Inheritance in Man (OMIM) which provides clinical descriptions of thousands of rare diseases. Second, we incorporate Immunoglobulin G (IgG) measurements from laboratory tests as a risk factor. We implement a joint analysis that leverages information across all features and labeled case data to compute a weight for each feature through a penalized regression framework. Comparing PheNet against the current state-of-the-art methods, we observe a 3-fold increase in the detection of true cases. We then conducted a retrospective analysis to assess the utility of PheNet as a predictive tool. We aggregated every patient’s EHR data in 30-day intervals, and computed each patient’s risk score using PheNet at each interval. We reviewed the labeled CVID cases who had a PheNet score in the 99.5th percentile at any point in time, and identified 21 patients whose score was in the top percentile before any billing code for immunodeficiency was present. As a clinical tool, our approach provides unprecedented information that can be utilized with minimal resources in hospitals and clinics that are far away from large academic medical centers.
[an error occurred while processing this directive]
I am currently a computer science PhD student at the University of California, Los Angeles (UCLA). I am advised by Sriram Sankararaman and Bogdan Pasaniuc. My current research involves developing scalable statistical methods for analyzing biobank-scale genomic datasets. Additionally, I am interested in machine learning models for electronic health record data, specifically for methods working to prioritize patients with rare diseases and eventually combining EHR and genomics studies. Previously, I have interned at Illumina and Sandia National Laboratories. Prior to graduate school, I earned my bachelor’s in Mathematics also at UCLA. My research is funded by a Eugene V. Cota-Robles Fellowship and NRT Grant.
[an error occurred while processing this directive]
Personal home page
[an error occurred while processing this directive]
[an error occurred while processing this directive]
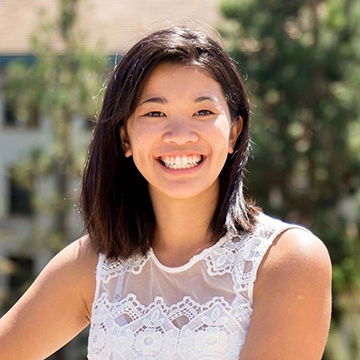