[an error occurred while processing this directive]
[an error occurred while processing this directive]
[an error occurred while processing this directive]
[an error occurred while processing this directive]
PhD Candidate
[an error occurred while processing this directive]
McGill University / Mila, Quebec
[an error occurred while processing this directive]
[an error occurred while processing this directive]
[an error occurred while processing this directive]
[an error occurred while processing this directive]
Artificial Intelligence
Control, Intelligent Systems, and Robotics
Theory
Reinforcement and Continual Learning
[an error occurred while processing this directive]
Bridging Perception and Action: Towards Continual Reinforcement Learning
[an error occurred while processing this directive]
My research goal is to develop Artificial Intelligence (AI) systems that can understand the data observed ("see"), learn to represent the world knowledge ("think''), and plan with acquired information ("act"), with the ability to adapt to changes, and become better learners over time. I tackle this problem by using reinforcement learning (RL), which allows agents to learn by trial-and-error, from interaction with their environment. My work aims to improve the applicability and scalability of reinforcement learning agents in real-world problems. Towards the goal of building machines that can see, think, and act, my research thus far has focused on the intersection of human visual attention (Khetarpal & Precup ICML18-LLARLA), representations of temporally abstract actions (Khetarpal & Precup AAAI19-DC, Khetarpal et al. AAAI20), and grounding learning in intuitive theories of affordances (Khetarpal et al. ICML20). I will talk about the key findings of my research so far including a framework that enables AI agents to ‘represent’ and ‘reason’ about their environment through the lens of affordances.
[an error occurred while processing this directive]
Khimya Khetarpal is a fourth-year Ph.D. candidate in the Reasoning and Learning Lab at McGill University and Mila, Montreal, advised by Doina Precup. She received a master’s degree in computer engineering from the University of Florida (UF) in 2016, and a bachelor’s degree in electronics and communication engineering from the Vellore Institute of Technology (VIT) in 2011. Prior to doctorate studies, She worked at the intersection of computer vision, and robotics at Intel, IIT Kanpur, and Robert Bosch. Her research interests span reinforcement learning, computer vision, and robotics, with a focus on developing artificial intelligence (AI) agents that can understand the data observed (“see”), learn to represent the world knowledge (“think”), and plan with acquired information (“act”), with the ability to adapt to changes and become better learners over time. She spent the Fall of 2019 interning at DeepMind Montreal. Her research has been featured in MIT Technology Review. Outside of research, She enjoys teaching and mentoring younger students through initiatives such as AI4Good Lab, Skype A Scientist, and FIRST Robotics.
[an error occurred while processing this directive]
Personal home page
[an error occurred while processing this directive]
[an error occurred while processing this directive]
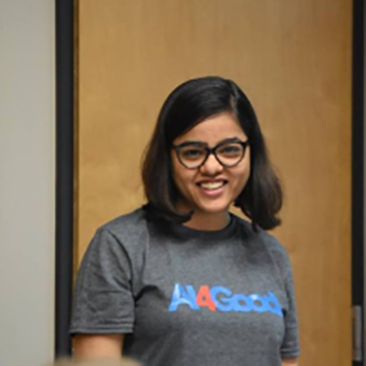