[an error occurred while processing this directive]
[an error occurred while processing this directive]
[an error occurred while processing this directive]
[an error occurred while processing this directive]
PhD Candidate
[an error occurred while processing this directive]
University of California, Berkeley
[an error occurred while processing this directive]
[an error occurred while processing this directive]
[an error occurred while processing this directive]
[an error occurred while processing this directive]
Artificial Intelligence
Signal Processing
Computational Imaging
[an error occurred while processing this directive]
Computational cameras for ML and ML for computational cameras
[an error occurred while processing this directive]
Intelligent systems often rely on information from cameras to reason about the world and make decisions, however conventional cameras are designed to take the most visually appealing images for humans rather than capture the most useful information for intelligent agents. Hyperspectral imaging can provide more information than color imaging for tasks such as tumor segmentation and crop monitoring, and 3D imaging can be useful for many robotics and biology applications. However, such imaging systems are often prohibitively costly and bulky. In my work, I utilize computational imaging, which is the co-design of optics and algorithms, to make very compact and cheap imagers that capture higher-dimensional information (3D and hyperspectral). I have demonstrated cameras that encode 3D information (e.g. hyperspectral reflectance) in a single measurement, then recover the encoded volume by solving a compressive-sensing-based inverse problem. These cameras can be incredibly small, often requiring no lenses or moving parts, and can be inexpensive to fabricate. While computational cameras could boost many machine learning tasks, machine learning methods can also be used to improve the reconstruction algorithms and the design of a computational camera itself. I have shown that it’s possible to combine our knowledge of the imaging system physics along with deep learning to create physics-based networks that solve the imaging inverse problem. These physics-based networks are faster than traditional approaches and require less training data than is needed by deep learning approaches (since we incorporate known physics into the network), enabling real-time image reconstructions. As more images are directly used in machine learning pipelines, it is increasingly important to have cameras that are optimized to capture relevant features for tasks instead of optimized for image quality based on human-perception. In my work, I aim to design computational cameras for better and more robust machine learning, as well as use machine learning to design better and more capable computational cameras.
[an error occurred while processing this directive]
Kristina Monakhova is a PhD candidate in UC Berkeley’s Electrical Engineering and Computer Sciences Department where she is a member of Laura Waller’s Computational Imaging research group. Her research focuses on making more capable cameras and microscopes through the co-design of imaging systems and algorithms. Her research lies at the intersection of signal processing, machine learning, and optics. Kristina received her Bachelor’s degree in Electrical Engineering from the State University of New York at Buffalo. She is a recipient of the NSF Graduate Research Fellowship and the NDSEG Fellowship. Outside of research, Kristina has a strong record of service and mentorship through leadership roles in the Electrical Engineering Graduate Student Association as well as the Women in Computer Science and Engineering (WICSE) at UC Berkeley.
[an error occurred while processing this directive]
Personal home page
[an error occurred while processing this directive]
[an error occurred while processing this directive]
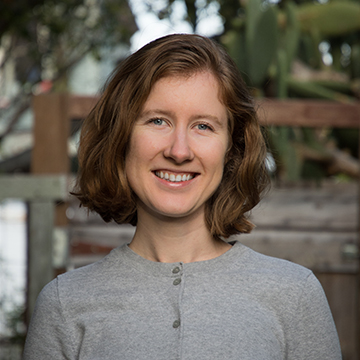