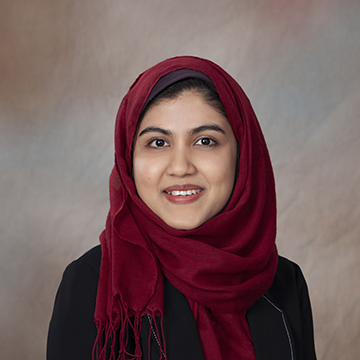
Postdoctoral Scholar
Carnegie Mellon University
PhD '20 University of Virginia
Areas of Interest
- Artificial Intelligence
- Control, Intelligent Systems, and Robotics
- Cyber-Physical Systems and Design Automation
- Human-Computer Interaction
Poster
EMSContExt: EMS Protocol-driven Concept Extraction for Cognitive Assistance in Emergency Response
Abstract
This poster presents a technique for automated curation of a domain-specific knowledge base or lexicon for resource-constrained domains, such as Emergency Medical Services (EMS), and its application to real-time concept extraction and cognitive assistance in emergency response. The EMS responders often verbalize critical information describing the situations at an incident scene, including patients’ physical condition and medical history. Automated extraction of EMS protocol-specific concepts from responders’ speech data can facilitate cognitive support by selecting and executing the proper EMS protocols for patient treatment. This task is similar to the traditional NLP task of concept extraction. However, the underlying application domain poses significant challenges, including low training resources availability (e.g., no existing EMS ontology, lexicon, or annotated EMS corpus) and domain mismatch.
Hence, I develop EMSContExt, a weakly-supervised concept extraction solution for EMS concepts. It utilizes different knowledge bases and a semantic concept model based on a corpus of over 9400 EMS narratives for lexicon expansion. The expanded EMS lexicon is then used to automatically extract critical EMS protocol-specific concepts from real-time EMS speech narratives. The experimental results show that EMSContExt achieves 0.85 recall and 0.82 F1-score for EMS concept extraction and significantly outperforms MetaMap, a state-of-the-art medical concept extraction tool. I also demonstrate the application of EMSContExt to EMS protocol selection and execution and real-time recommendation of protocol-specific interventions to the EMS responders. Here, EMSContExt outperforms MetaMap with a 6% increase and six times speedup in weighted recall and execution time, respectively.
Bio
Sarah Preum is a postdoctoral fellow in the School of Computer Science at Carnegie Mellon University. At CMU, she works with Professor John Zimmerman to provide data-driven clinical decision support in the Intensive Care Unit. Sarah has developed novel natural language processing, knowledge integration, and temporal modeling techniques to provide personalized decision support for health safety. Some of her major research projects include (i) multi-modal conflict detection and prediction for assistive health applications, (ii) identifying unsafe health information, and (iii) developing wearable intelligent assistant to provide real-time decision support to emergency responders. Sarah has received her Ph.D. in CS from the University of Virginia under the supervision of Professor John A. Stankovic.
Sarah is a recipient of the UVA Graduate Commonwealth Fellowship, the Adobe Research Graduate Scholarship, the NSF Smart and Connected Health Student Award, and the UVA Big Data Fellowship. Her work has been published in premier CS conference proceedings and journals, including ICDE, CIKM, PerCom, IPSN, ACM CSUR, and IEEE Trans. of CPS.