[an error occurred while processing this directive]
[an error occurred while processing this directive]
[an error occurred while processing this directive]
[an error occurred while processing this directive]
PhD Candidate
[an error occurred while processing this directive]
Stanford University
[an error occurred while processing this directive]
[an error occurred while processing this directive]
[an error occurred while processing this directive]
[an error occurred while processing this directive]
Artificial Intelligence
[an error occurred while processing this directive]
Surprises in Robust Machine Learning
[an error occurred while processing this directive]
Standard machine learning produces models that are highly accurate on average but that degrade dramatically when the test distribution deviates from the training distribution. While one can train robust models, this often comes at the expense of standard accuracy (on the training distribution). We study this tradeoff in two settings: adversarial training to be robust to perturbations and upweighting minority groups to be robust to subpopulation shifts. We create simple examples which highlight generalization issues as a major source of this tradeoff. For adversarial examples, we show that even augmenting with correctly annotated data to promote robustness can produce less accurate models, but we develop a simple method, robust self-training, that mitigates this tradeoff using unlabeled data. For minority groups, we show that overparametrization of models can hurt accuracy on the minority groups, though it improves standard accuracy. These results suggest that the "more data" and "bigger models" strategy that works well for the standard setting where train and test distributions are close, need not work on out-of-domain settings.
[an error occurred while processing this directive]
Aditi Raghunathan is a fifth year PhD student at Stanford University advised by Percy Liang. She is interested in building robust ML systems that can be deployed in the wild. She is a recipient of the Open Philanthropy AI Fellowship and the Google PhD fellowship in Machine Learning.
[an error occurred while processing this directive]
Personal home page
[an error occurred while processing this directive]
[an error occurred while processing this directive]
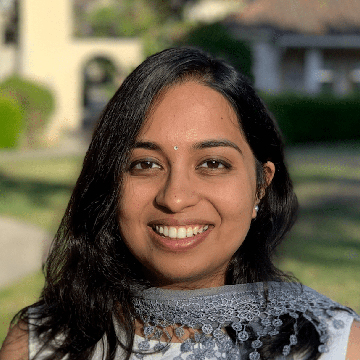