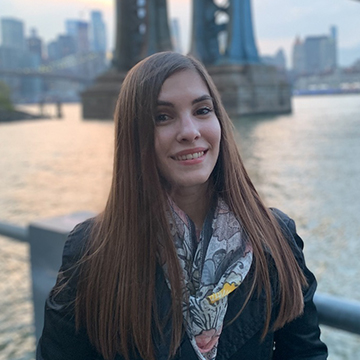
PhD Candidate
New York University
Areas of Interest
- Artificial Intelligence
- Reinforcement Learning
Poster
Fast Adaptation to New Environments via Policy-Dynamics Value Functions
Abstract
Standard RL algorithms assume fixed environment dynamics and require a significant amount of interaction to adapt to new environments. We introduce Policy-Dynamics Value Functions (PD-VF), a novel approach for rapidly adapting to dynamics different from those previously seen in training. PD-VF explicitly estimates the cumulative reward in a space of policies and environments. An ensemble of conventional RL policies is used to gather experience on training environments, from which embeddings of both policies and environments can be learned. Then, a value function conditioned on both embeddings is trained. At test time, a few actions are sufficient to infer the environment embedding, enabling a policy to be selected by maximizing the learned value function (which requires no additional environment interaction). We show that our method can rapidly adapt to new dynamics on a set of MuJoCo domains.
Bio
I am a PhD student in computer science at NYU, advised by Rob Fergus as part of the CILVR lab. My research focuses on deep reinforcement learning. Previously, I got my B.A. in Astrophysics from Princeton University, where I worked with Michael Strauss on theoretical cosmology and Eve Ostriker on supernovae simulations. I also did research internships at Facebook AI Research and Microsoft Research.
I am interested in designing machine learning algorithms that can make robust sequential decisions in complex environments. My research spans various problems in reinforcement learning including exploration, fast adaptation to new environments, multi-agent and transfer learning. My current focus is on understanding and improving the generalization and robustness of reinforcement learning agents.