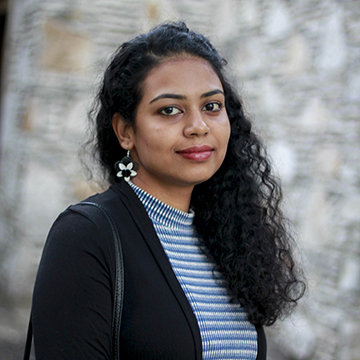
PhD Candidate
University of Massachusetts Amherst
Areas of Interest
- Artificial Intelligence
- Control, Intelligent Systems, and Robotics
Poster
Reliable Decision-Making with Incomplete Knowledge
Abstract
Artificial intelligence (AI) systems are increasingly deployed in a wide variety of settings and have broad societal impacts. Evaluating these systems using aggregate measures of accuracy hides important shortcomings. Systems that demonstrate promising results in structured environments may sometimes produce unexpected and undesirable consequences, when deployed. The severity of the undesirable behavior ranges from mild and tolerable events to safety-critical failures. Reliable decision-making is critical in high-impact domains such as self-driving cars and health care robots. My research focuses on improving the reliability of AI systems by minimizing their undesirable behavior. In particular, I develop techniques for reliable decision-making when the system operates based on incomplete knowledge of the environment in which it is situated. I characterize the different types of negative side effects of a deployed AI system and develop techniques to minimize the undesirable impacts, without entirely redesigning its model or suspending its operation. Additionally, I present techniques to identify regions in the model where the system is more prone to make mistakes. To facilitate effective failure analysis and for the user to determine when to trust the system, I develop methods to improve the interpretability of the generated solutions.
Bio
Sandhya Saisubramanian is a Ph.D. candidate at the University of Massachusetts, Amherst, advised by Shlomo Zilberstein. Her research interests are in developing techniques to improve the reliability of AI systems that operate in complex real-world settings. She earned her M.S. in computer science from the National University of Singapore, Singapore, and her B.Tech. in computer science from the Puducherry Technological University, India.