[an error occurred while processing this directive]
[an error occurred while processing this directive]
[an error occurred while processing this directive]
[an error occurred while processing this directive]
PhD Candidate
[an error occurred while processing this directive]
University of California, Berkeley
[an error occurred while processing this directive]
[an error occurred while processing this directive]
[an error occurred while processing this directive]
[an error occurred while processing this directive]
Artificial Intelligence
Control, Intelligent Systems, and Robotics
[an error occurred while processing this directive]
Last-Mile Delivery of Computer Vision with Test-Time Adaptation
[an error occurred while processing this directive]
Immense data and gigantic models have been the driving force for the tremendous success of AI technologies. However, many real-world applications simply do not have access to large scale labeled data or the computing power to support large models. In this work, I will talk about the “last-mile delivery” problem of computer vision, where the goal is to build models that are adapted to any test scenario with limited data, annotation, and computation. The thesis of our approaches is test-time adaptation where the model learns to utilize the test-time information to adjust its parameters and structures. We test our methods on few-shot detection, zero-shot learning, and image classification. The results show that test-time adaptation can achieve state-of-the-art results in those problems and great computation reduction.
[an error occurred while processing this directive]
Xin Wang is a Ph.D. candidate in Computer Science at UC Berkeley, advised by Prof. Joseph E. Gonzalez and Prof.Trevor Darrell. She is a member of the Berkeley AI Research (BAIR) Lab, RISE Lab, and Berkeley Deep Drive. Before UC Berkeley, she obtained her B.S. degree in Computer Science from Shanghai Jiao Tong University in 2015. Her research interest lies in computer vision and machine learning, including dynamic representation frameworks for low shot learning, continual learning, efficient inference with applications in autonomous driving, and robotics. She has served as a co-organizer of the women in computer vision (WiCV) workshop in ECCV 2020 and the human in the loop (HILL) workshop at ICML 2019 and 2020.
[an error occurred while processing this directive]
Personal home page
[an error occurred while processing this directive]
[an error occurred while processing this directive]
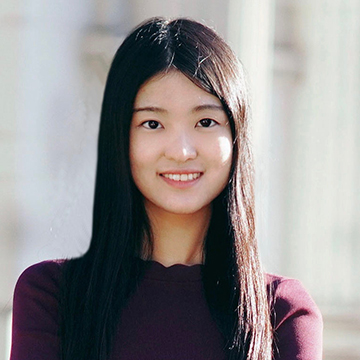