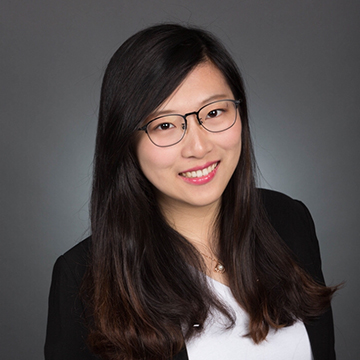
Importantly, for our learning dynamics, a fixed point belief can incorrectly estimate the payoffs of strategies that are different from the corresponding fixed point strategy. Thus, the learning dynamics may not converge to a complete information Nash equilibrium. We provide a sufficient and necessary condition under which the fixed point belief must have complete information of the unknown parameter. We also provide a sufficient condition which ensures that the fixed point strategy is a complete information equilibrium even when the parameter learning is incomplete.
Finally, we apply our results to Bayesian learning in congestion games with unknown latency functions. We provide two specific results for this setting: (i) Conditions under which strategic agents learn towards an equilibrium routing strategy profile with complete information of cost parameter; (ii) An adaptive tolling mechanism that eventually induces the socially optimal outcome.
Authors: Manxi Wu, Saurabh Amin, Asu Ozdaglar. [an error occurred while processing this directive] Manxi Wu is a doctoral student in the Social and Engineering Systems program in the Institute for Data, Systems and Society (IDSS) at MIT. Previously, she obtained B.S. in Applied Math from Peking University (2015) and M.S. in Transportation from MIT (2017). She focuses on developing system-theoretic tools for the efficiency and resiliency of urban systems. In her PhD research, she is developing models for strategy learning, information design, and pricing mechanisms, with the goal of improve socially desirable outcomes even under system disruptions. Manxi is a 2021 Siebel Scholar. She was awarded MIT IDSS Hammer Fellow in 2018. Her master thesis won Council of University Transportation Center’s Milton Pikarsky Memorial Award in 2017. [an error occurred while processing this directive] [an error occurred while processing this directive] [an error occurred while processing this directive]