[an error occurred while processing this directive]
[an error occurred while processing this directive]
[an error occurred while processing this directive]
[an error occurred while processing this directive]
PhD Candidate
[an error occurred while processing this directive]
University of Washington
[an error occurred while processing this directive]
[an error occurred while processing this directive]
[an error occurred while processing this directive]
[an error occurred while processing this directive]
Artificial Intelligence
Human-Computer Interaction
Natural Language Processing
[an error occurred while processing this directive]
Principles and Interactive Tools for Evaluating and Improving the Behavior of NLP models
[an error occurred while processing this directive]
While the accuracy of Natural Language Processing (NLP) models has been going up, users have more expectations than captured by just accuracy. Despite practitioners' attempt to inspect model blind spots or lacking capabilities, the status-quo processes can be ad-hoc and biased. My thesis focuses on helping practitioners organize and explore the inputs and outputs of their models, such that they can gain more systematic insights into their models' behaviors. I identified two building blocks that are essential for informative analysis: (1) to scale up the analysis by grouping similar instances, and (2) to isolate important components by generating counterfactuals. To support multiple analysis stages (training data assessment, error analysis, model testing), I designed various interactive tools that instantiate these two building blocks. In the process, I characterized the design space of grouping and counterfactual generation, seeking to balance the machine powers and practitioners' domain expertise. My future work proposes to explore how the grouping and counterfactual techniques can benefit non-experts in the data collection process.
[an error occurred while processing this directive]
I am a fifth year Computer Science PhD student at the University of Washington (UW-Seattle). My co-advisors are Jeffrey Heer from the Interactive Data Lab and Dan Weld from the Lab for Human-AI Interaction (HAI). I received my B.Eng from the Hong Kong university of Science and Technology (HKUST), majoring in Computer Science and Engineering. During my undergraduate studies, I was a member of the VisLab, and was supervised by Prof. Huamin Qu. I am particularly interested in Human Computer Interaction, Natural Language Processing, and Visualization. Currently, my work focuses on enhancing the training and evaluation steps of NLP models with the spirit of human-in-the-loop, such that users can more effectively and systematically interact with their models.
[an error occurred while processing this directive]
Personal home page
[an error occurred while processing this directive]
[an error occurred while processing this directive]
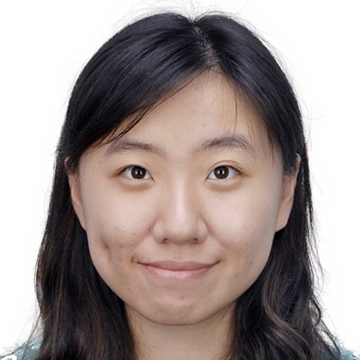