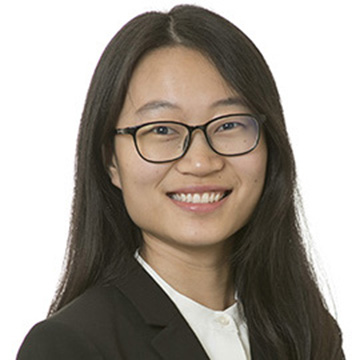
This presentation will highlight our recent efforts to make robust statistical inference by combining techniques from both statistics and optimization. In particular, the first part of the presentation will focus on two theoretical developments: one is the sequential change detection for multi-sensors, assuming the change happens to the covariance structure of the sensor network; the other is robust hypothesis testing that hedge against the distributional uncertainties in data-driven scenario. The second part of this presentation will provide a variety of applications of sequential change detection, such as seismic tremor detection human activity detection, as well as new opportunities and challenges in this field. [an error occurred while processing this directive] Liyan Xie is a fifth-year Ph.D. Candidate at Georgia Institute of Technology in the H. Milton Stewart School of Industrial and Systems Engineering. She received her B.Sc. in Statistics from University of Science and Technology of China (USTC) in 2016. Her research interests are the intersection between statistics and optimization, with a primary focus on sequential change detection and their applications in sensor networks and industrial quality control. She has worked on sequential detection, robust hypothesis testing, and spatio-temporal modeling in seismic data analysis and wearable sensors. She was awarded the finalist of INFORMS QSR (Quality, Statistics, and Reliability) student paper competition and Runner-up for the INFORMS Computing Society Student Paper Prize in 2019. She received the 2020 ARC student fellowship and IDEaS-TRIAD Research Scholarships from the Transdisciplinary Research Institute for Advancing Data Science (TRIAD) at Georgia Tech. [an error occurred while processing this directive] Personal home page [an error occurred while processing this directive] [an error occurred while processing this directive]