[an error occurred while processing this directive]
[an error occurred while processing this directive]
[an error occurred while processing this directive]
[an error occurred while processing this directive]
Postdoctoral Scholar
[an error occurred while processing this directive]
University of California, Berkeley
[an error occurred while processing this directive]
PhD '18 Boston University
[an error occurred while processing this directive]
[an error occurred while processing this directive]
[an error occurred while processing this directive]
Artificial Intelligence
Computer Vision
Deep Learning
Natural Language Processing
[an error occurred while processing this directive]
Compositional and Robust Action Understanding
[an error occurred while processing this directive]
In the era with massive video data from a wide range of applications (e.g. smart home, medical instrument and smart transportation, etc.), designing algorithms to understand action and promote machines to act as human behavior will greatly benefit our life, yet current video understanding algorithms are far from achieving the level of human intelligence. Early video understanding technologies mostly focus on fully supervised trimmed action recognition using holistic frame modeling without reasoning capability. On the one hand, encoding the whole video scene in a holistic way with mixed subject, object and background will introduce background bias and cause the action recognition model to be brittle to different contexts. Understanding action in a compositional way with localized foreground subjects/objects can help reduce confounding variables and possibly bridge the connection with the common affordance knowledge of involved objects empowering the model reasoning ability. On the other hand, in practical applications, continuous video streams require to temporally localize actions before trimmed action recognition, yet such annotation is expensive and suffers from annotation consistency issues as well as inadequate description using only action labels. To overcome these issues, one possible direction is detecting action with less supervision and incorporating language to describe actions. In summary, the ultimate goal of my research is building robust action understanding algorithms with human level structural knowledge and multi-modal complementary ability. To achieve this goal, my research touches the following two aspects: (1) compositional action recognition with knowledge reasoning, and (2) multimodal action detection with less supervision.
[an error occurred while processing this directive]
She is a postdoctoral scholar in the EECS department at UC Berkeley advised by Prof. Trevor Darrell. She received her PhD degree from the computer science department at Boston University in 2018 advised by Prof. Kate Saenko. Her research focuses on deep learning, computer vision and natural language processing, particularly in the area of action understanding in video. Her R-C3D work has received the Most Innovative Award in ActivityNet Challenge 2017. She interned at Disney Research, Pittsburgh with Prof. Leonid Sigal.
[an error occurred while processing this directive]
Personal home page
[an error occurred while processing this directive]
[an error occurred while processing this directive]
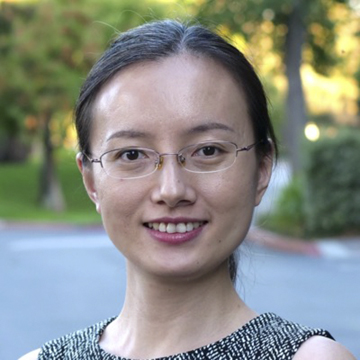