[an error occurred while processing this directive]
[an error occurred while processing this directive]
[an error occurred while processing this directive]
[an error occurred while processing this directive]
PhD Candidate
[an error occurred while processing this directive]
McGill University / Mila Institute, Quebec
[an error occurred while processing this directive]
[an error occurred while processing this directive]
[an error occurred while processing this directive]
[an error occurred while processing this directive]
Artificial Intelligence
Control, Intelligent Systems, and Robotics
Reinforcement Learning
[an error occurred while processing this directive]
Learning Invariant Representations for Reinforcement Learning without Reconstruction
[an error occurred while processing this directive]
We study how representation learning can accelerate reinforcement learning from rich observations, such as images, without relying either on domain knowledge or pixel-reconstruction. Our goal is to learn representations that both provide for effective downstream control and invariance to task-irrelevant details. Bisimulation metrics quantify behavioral similarity between states in continuous MDPs, which we propose using to learn robust latent representations which encode only the task-relevant information from observations. Our method trains encoders such that distances in latent space equal bisimulation distances in state space. We demonstrate the effectiveness of our method at disregarding task-irrelevant information using modified visual MuJoCo tasks, where the background is replaced with moving distractors and natural videos, while achieving SOTA performance. We also test a first-person highway driving task where our method learns invariance to clouds, weather, and time of day. Finally, we provide generalization results drawn from properties of bisimulation metrics, and links to causal inference.
[an error occurred while processing this directive]
I am a final year PhD candidate at McGill University and the Mila Institute, co-supervised by Joelle Pineau and Doina Precup. I work on state abstractions and learning representations for generalization in reinforcement learning. I have an M.Eng. in EECS and dual B.Sci. degrees in Mathematics and EECS from MIT.
[an error occurred while processing this directive]
Personal home page
[an error occurred while processing this directive]
[an error occurred while processing this directive]
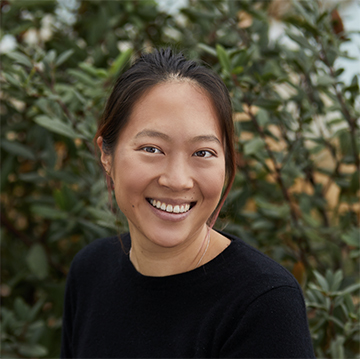